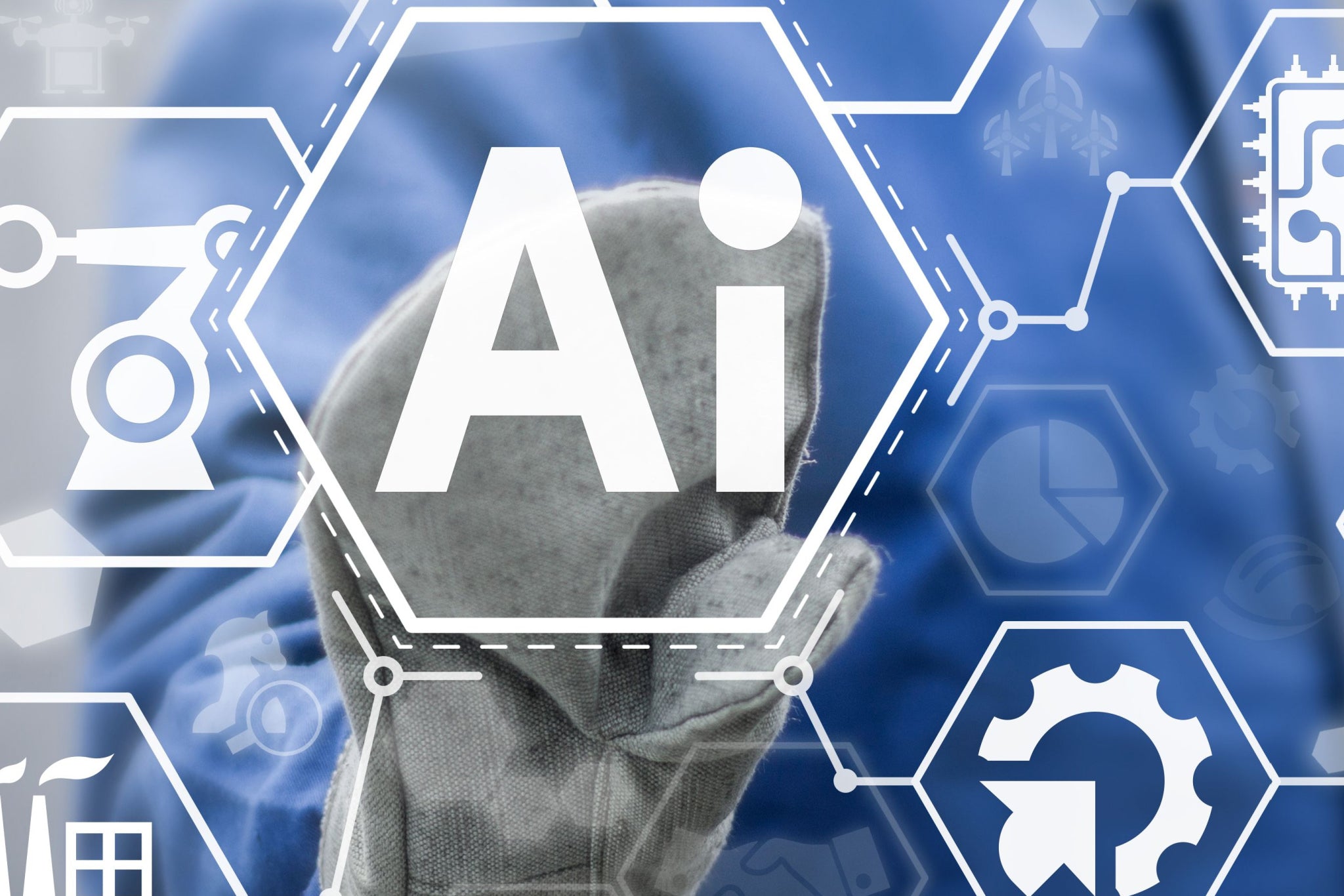
SEMI102 Artificial Intelligence for Thin Film Manufacturing
This course describes how machine learning and AI-based approaches to research, development, and production brings advantages to cleanroom processes. AI-based identification of time-varying equipment performance, and the effects of the previous recipe used on the outcome of the current desired methods, are just some of the ways AI can be used to reduce the process variability.
Originally recorded August, 2020, this course is now offered in eLearning format.
SEMI Standards PV and PV Materials China Joint TC Chapter Spring Meeting 2020
This course describes how machine learning and AI-based approaches to research, development, and production brings advantages to cleanroom processes. AI-based identification of time-varying equipment performance, and the effects of the previous recipe used on the outcome of the current desired methods, are just some of the ways AI can be used to reduce the process variability.
The team from Cornell has applied AI approaches to optimize lithography and etching processes involved in the development of an RF wake-up NEMS (Nano ElectroMechnical system) switch that needs a well-controlled gap between a moving shuttle and a contact. We report on a decision tree based AI model for predicting lithography outcomes.
This work is being applied to plasma etching and the combined prediction of lithography and thin-film etching, using CD-SEM imagery for feature extraction and modeling process variables. Additional approaches to train process-modeling CAD tools to result in better process development experience are developed.
Originally recorded August, 2020, this course is now offered in eLearning format.
SEMI Standards PV and PV Materials China Joint TC Chapter Spring Meeting 2020
This course describes how machine learning and AI-based approaches to research, development, and production brings advantages to cleanroom processes. AI-based identification of time-varying equipment performance, and the effects of the previous recipe used on the outcome of the current desired methods, are just some of the ways AI can be used to reduce the process variability.
The team from Cornell has applied AI approaches to optimize lithography and etching processes involved in the development of an RF wake-up NEMS (Nano ElectroMechnical system) switch that needs a well-controlled gap between a moving shuttle and a contact. We report on a decision tree based AI model for predicting lithography outcomes.
This work is being applied to plasma etching and the combined prediction of lithography and thin-film etching, using CD-SEM imagery for feature extraction and modeling process variables. Additional approaches to train process-modeling CAD tools to result in better process development experience are developed.
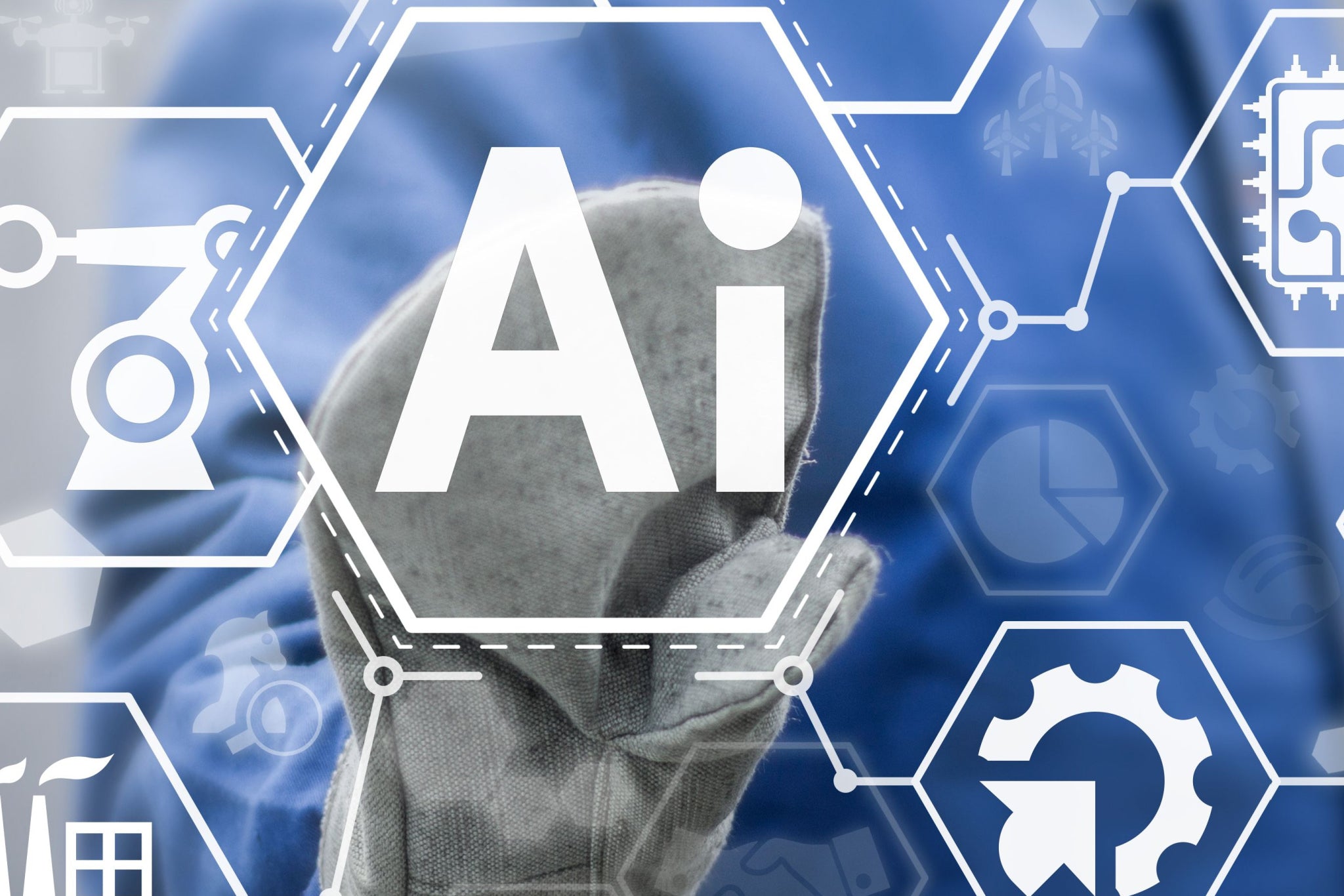
SEMI102 Artificial Intelligence for Thin Film Manufacturing
Sale price$49.00 USD
Regular price$49.00 USD (/)
This product has no reviews yet.